The Importance of Data Labeling in Business
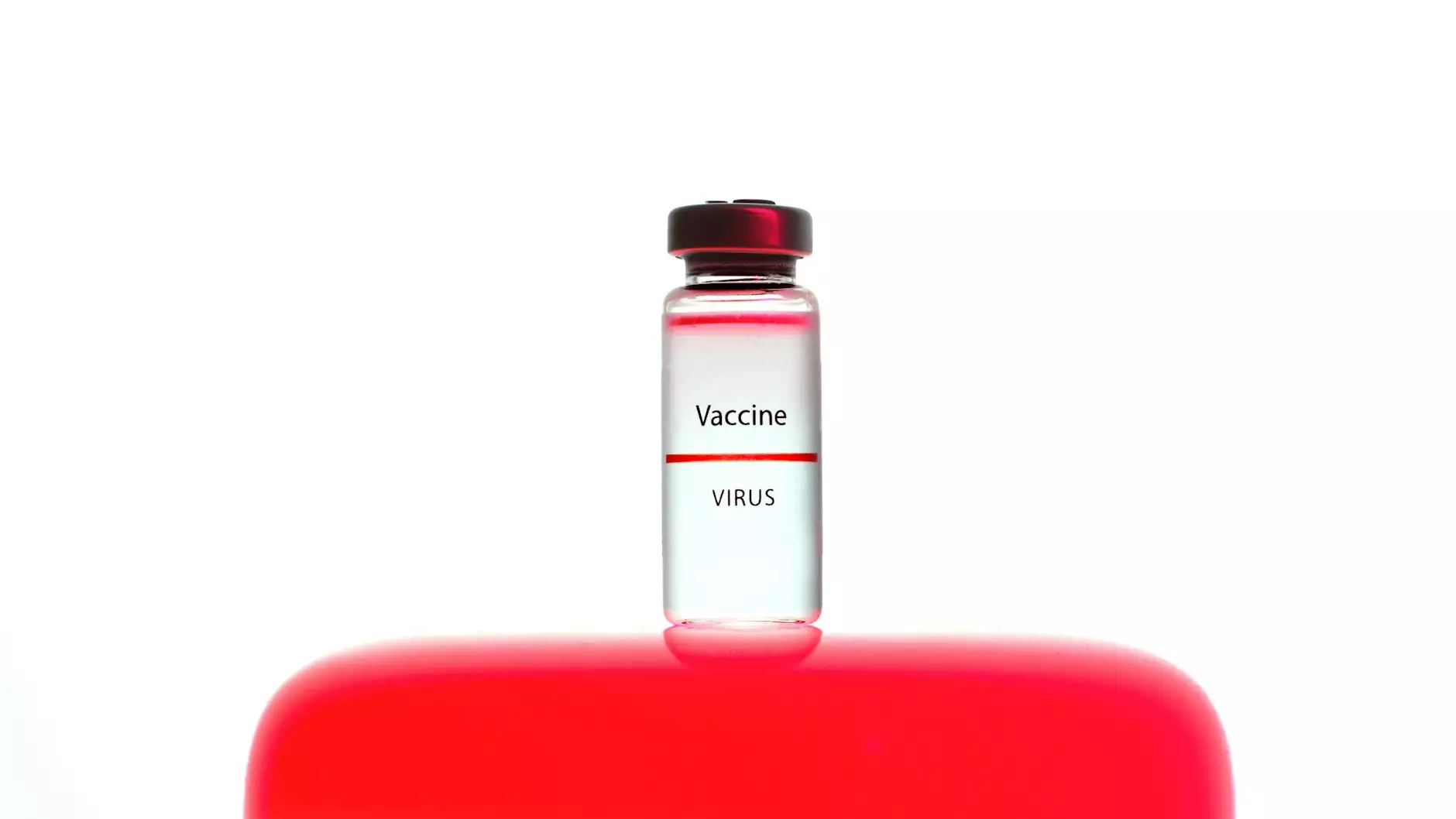
In the rapidly evolving landscape of modern business, the need for accurate and effective data labeling has become more prominent than ever. Industries are increasingly relying on data-driven decision-making, and the quality of this data often hinges on the effectiveness of the labeling processes employed. This article delves into the significance of data labeling, particularly in sectors like Home Services and Keys & Locksmiths, and how it can elevate the performance of businesses such as keymakr.com.
What is Data Labeling?
Data labeling is the process of identifying and tagging data points in datasets, enabling machines to understand and categorize information for various applications, particularly in machine learning (ML) and artificial intelligence (AI). This process is essential in training algorithms, allowing them to make accurate predictions and classifications based on the labeled input they receive.
Types of Data Labeling
- Image Annotation: Tagging images to classify or identify objects within them.
- Text Classification: Assigning tags or categories to pieces of text based on content.
- Audio Labeling: Annotating audio clips to recognize speech patterns, sounds, or other auditory data.
- Video Annotation: Tagging frames of video to identify movements or specific events that occur over time.
Why is Data Labeling Essential for Businesses?
In today's competitive market, businesses cannot afford to overlook the importance of data labeling. Here are several reasons why it is vital:
1. Enhances Machine Learning Models
Machine learning algorithms require large amounts of labeled data to learn and improve. Proper data labeling ensures that algorithms can recognize patterns, which is crucial for tasks such as predictive analytics and automation. By providing correctly labeled datasets, businesses can enhance the accuracy and efficacy of their AI systems.
2. Streamlines Operations
For companies in the Home Services and Keys & Locksmiths sectors, having access to well-labeled data can significantly streamline operations. For example, effective labeling of customer service inquiries can enable automated responses and categorization, reducing wait times and improving service delivery.
3. Improves Customer Experience
A well-structured data labeling system can analyze customer feedback, preferences, and behaviors. This analysis enables businesses to tailor their services, leading to enhanced customer satisfaction. In industries such as locksmith services, where quick responses are paramount, leveraging labeled data can create a responsive and personalized experience.
Data Labeling Techniques and Tools
With the increasing demand for data labeling, various techniques and tools have emerged to assist businesses in this crucial area. Here are some popular approaches:
1. Manual Labeling
This traditional method involves human beings labeling data manually. While it can be time-consuming and prone to error, it is often necessary for complex datasets that require nuanced understanding.
2. Automated Labeling Software
Automation tools can significantly reduce the time and effort required for data labeling. These tools use pre-trained AI models to accelerate the labeling process. However, they may need human oversight to ensure accuracy.
3. Crowdsourced Labeling
Crowdsourcing involves leveraging the power of a large group of people to label data. This method can provide diverse perspectives and can be particularly effective for large datasets. Platforms like Amazon Mechanical Turk offer such services.
Challenges in Data Labeling
Despite its importance, data labeling comes with several challenges:
1. Quality Control
Ensuring the quality of labeled data is a significant challenge. If the data is incorrectly labeled, the models trained on it may perform poorly, leading to flawed business decisions.
2. Time Consumption
Manual labeling can be incredibly time-consuming, especially with large datasets. Companies need to balance the speed of labeling with the accuracy of results.
3. Cost Implications
High-quality data labeling can be expensive, especially if done manually or through crowdsourcing. Budgeting for these expenses while ensuring product quality is a common hurdle.